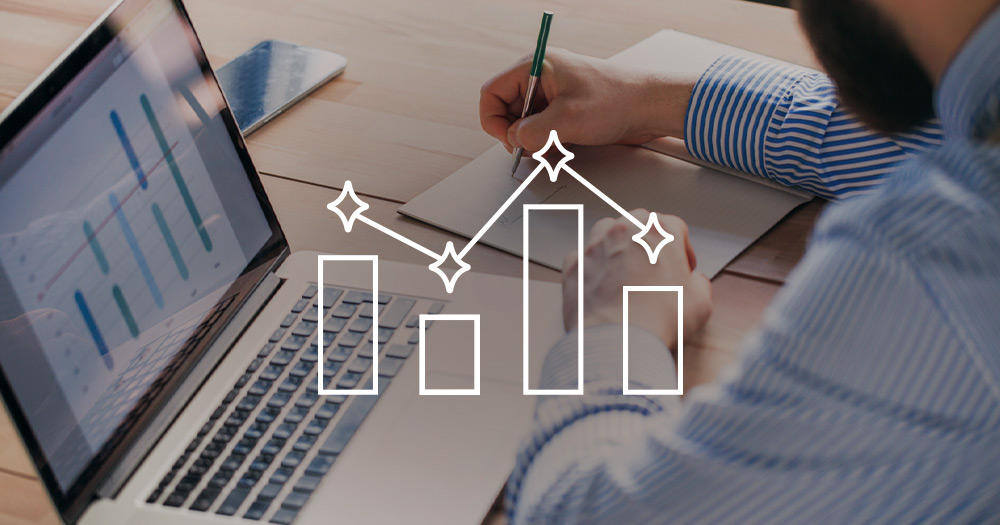
4 Steps to Make Your Data Dazzle
How many times have you heard a brand exclaim they are data-driven? It’s a phrase that many brands are using, but what goes into the data that drives them? Why are those brands so confident in their data-driven decisions? Below are a few considerations regarding data cleaning and data analysis when leveraging data for your brand.
Accuracy
Accurate data is important if you are to gain any actionable insights from your study. Your data could be giving you a clear answer, but if your data is wrong then your answer is likely wrong, too. One reason your data might not be accurate is inattentive respondents. Some respondents may zoom through your study without paying much attention to what you’re asking. Most survey software records the amount of time it takes participants to complete your survey. You can examine the fastest 5% or 10% of response times to determine if those times are reasonable or not. If they seem too fast, it may be worth removing those individuals from your analyses. Additionally, respondents may select the same answer choice on all your questions (i.e., straightlining). Removing these individuals leaves you with more accurate data.
Another way to check for accuracy is to examine open-ended responses. If it’s a simple typo, it may not be worrisome. However, some respondents produce useless data, such as when they “keyboard smash” and enter a gibberish response. Be cautious of these individuals. We should note these approaches are often used in combination, too. A respondent may have a quick response time that’s believable, but then you discover they have straightlined most of their responses. These steps may seem subjective, but that’s because they are. The important thing to remember is to keep your removal process standardized so everyone meets the same criteria. Unlike Oscar the Grouch, we as researchers don’t like garbage. These steps help to get rid of the garbage and leave you with more accurate data.
Properly Formatted
Next, take the time to ensure your data is programmed and coded correctly. For example, if you’re gathering frequency data, code the values and the answers as the same. If a respondent says they have purchased a product 5+ times, code their response as a 5, not as a 1. Similarly, if conducting a tracking study, make sure your answer options are coded the same between waves. This is less error prone and can prevent unnecessary headaches (that is if you catch your mistake, otherwise your data is just plain wrong). Also, think about how your survey is programmed. Are you taking steps to reduce potential biases in the data, such as randomizing the order in which responses appear? Are you avoiding leading (e.g., “Do you agree that our brand is great?”) and double-barreled (e.g., “Do you think our products are high quality and affordable?”) questions? Some survey software allows you to test your survey before fielding and will alert you to many programming errors (such as terminating responses at the wrong time), although this isn’t perfect. Sometimes you can even download the test data to make sure the data is set up correctly before you field.
Complete
You should aim to have as many people as possible answer every question you’re asking. This helps provide an adequate sample size for all analyses you may want to perform. Having a large sample allows for more statistical power, which increases the likelihood of finding a difference if one exists. When constructing your survey, you should pay close attention to its length and complexity. Longer surveys require you to hold respondents’ attention for longer, and that can lead to bad data. A standard rule of thumb is to create a survey that takes 10 to 15 minutes to complete. Qualtrics suggests keeping surveys under 12 minutes.1 If your survey needs to be longer than this, keep in mind it may take more time to obtain an adequate sample.
After fielding, you can check sample sizes to ensure the survey acted the way you wanted it to. For example, if your survey was taken by people who have either a full-time or part-time job, but you ask a question about job satisfaction only to people who are employed full time, then the sample size for that question should be lower than your overall sample. It’s much easier to compare answers with complete data and it means you don’t have to fill in the blanks. Otherwise, you may have to impute scores (e.g., mean or median imputation, which could be the topic of another blog), which has several drawbacks, or go without using those who did not provide a response and lower your sample size.
Useful
Your goal isn’t to rattle off every statistic from every analysis you ran (you’re not an auctioneer). Instead, think about how your analyses answered the research question. Does the data you’re describing add value to your brand? Is it something your brand can use to improve? You should focus on telling a story with your data. Provide findings that help stakeholders better understand their brand, their target and how to reach that target.
Not quite sure how to put these techniques to use? Contact us and we can help you leverage good data for your brand.